The Importance of Agriculture Datasets for Machine Learning in Modern Business
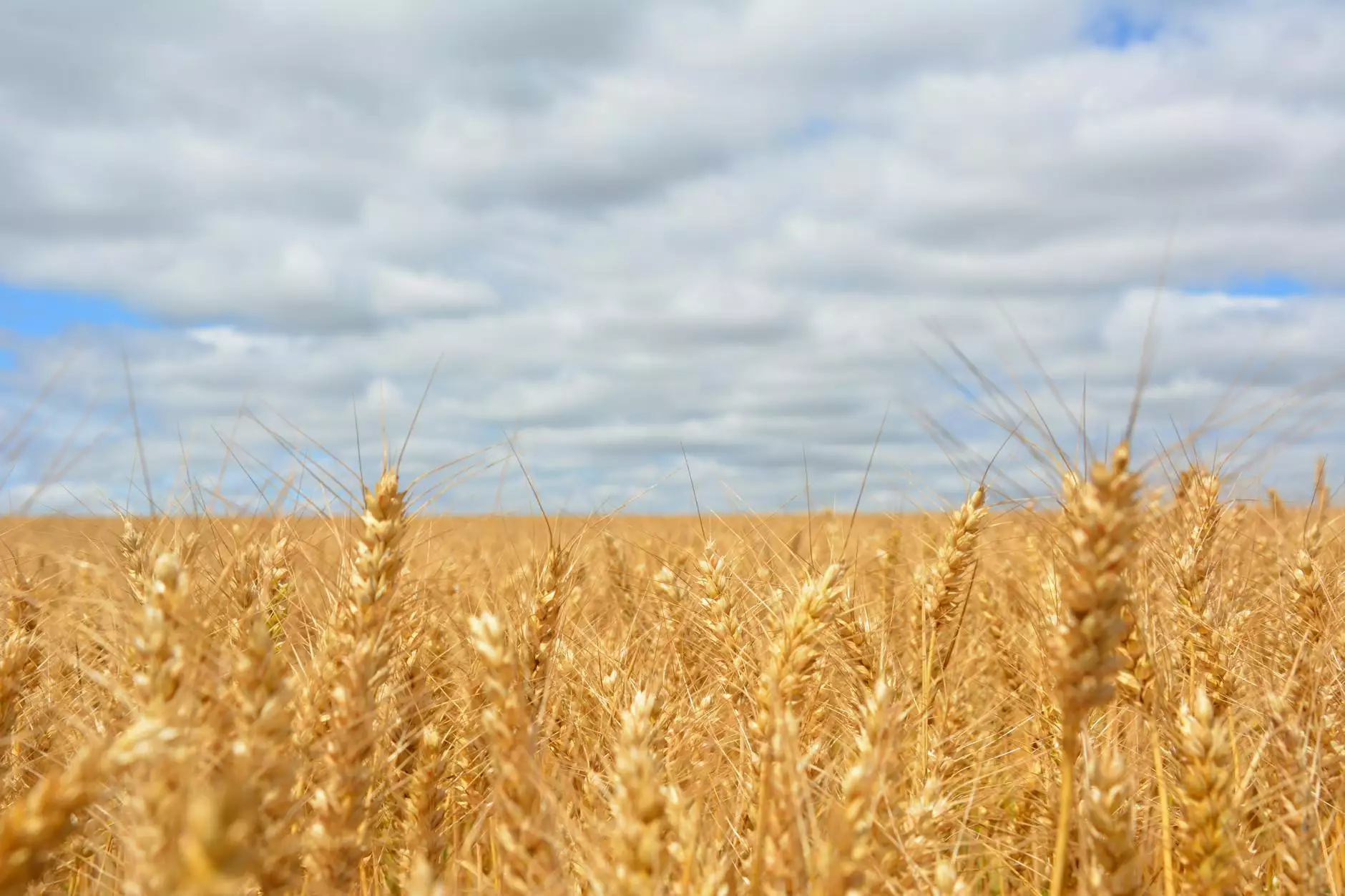
In today's world, the intersection of technology and agriculture is transforming the way we approach farming business. One of the most significant advancements in this area is the use of agriculture datasets for machine learning. As businesses increasingly adopt sophisticated technological solutions, the utilization of data-driven methodologies has become essential for gaining competitive advantages.
Understanding Agriculture Datasets
Agriculture datasets are collections of data specifically related to farming activities, environmental conditions, crop yields, soil properties, and more. These datasets can vary in size and complexity and can be structured or unstructured. In the context of machine learning, structured data is particularly valuable as it can easily be processed and analyzed.
The Role of Machine Learning in Agriculture
Machine learning (ML) is a subset of artificial intelligence that focuses on the development of algorithms that can learn from and make predictions based on data. In agriculture, machine learning is used for various applications, including:
- Predictive Modeling: Utilizing historical data to forecast crop yields or monitor pest outbreaks.
- Precision Farming: Optimizing resources, such as water and fertilizers, based on real-time data analysis.
- Automated Crop Surveillance: Leveraging drones and satellite imagery to gather data on crop health.
- Supply Chain Optimization: Enhancing the efficiency of the agricultural supply chain through data analysis.
Why Are Agriculture Datasets Critical for Machine Learning?
The effectiveness of machine learning models heavily relies on the quality and diversity of the underlying data. Here’s why agriculture datasets for machine learning are crucial:
- Rich Insights: Comprehensive datasets provide agriculture specialists with insights that can lead to better decision-making processes.
- Training High-Quality Models: Diverse datasets enable the development of robust ML models that can adapt to varying conditions and circumstances.
- Identifying Trends and Patterns: These datasets allow analysts to identify trends over time, helping to predict market shifts and consumer demands.
- Enhancing Sustainability: By analyzing data, businesses can develop sustainable practices that minimize environmental impact.
Sources of Agriculture Data
The data needed for effective machine learning applications in agriculture can be sourced from several places:
- Satellite Imagery: Satellites provide valuable data on land use, crop health, and weather patterns.
- Weather Stations: Real-time weather data can greatly affect crop yield predictions.
- Farm Equipment Sensors: Modern tractors and harvesters are equipped with sensors that collect real-time data on soil conditions and crop growth.
- Government and Research Institutions: Many governmental agencies provide publicly available datasets for research purposes.
Case Studies of Successful Implementations
Numerous businesses have successfully integrated agriculture datasets for machine learning to improve their operations:
Case Study 1: Blue River Technology
Blue River Technology developed a system called "See & Spray," which uses computer vision and machine learning to precisely identify weeds in cotton fields and apply herbicides only where necessary. This innovation has increased yield while significantly reducing chemical usage.
Case Study 2: Subtle Medical
Though focused on healthcare, Subtle Medical's techniques have implications in agricultural imaging. They developed AI algorithms that enhance imaging data for better analysis, which could equally apply to agricultural satellite imagery, improving productivity insights.
Challenges in Using Agriculture Datasets
While the benefits of employing agriculture datasets for machine learning are significant, several challenges remain:
- Data Quality: The accuracy of insights derived from machine learning models depends on the quality of the datasets used.
- Data Privacy: Ethical considerations relating to farmers’ data can impede data-sharing initiatives.
- Integration Issues: Combining data from various sources can be technically challenging and requires robust infrastructure.
Future Trends in Agriculture Data and Machine Learning
The future of agriculture is heavily tied to advancements in technology. Some emerging trends include:
- Increased Use of IoT in Agriculture: The integration of IoT devices will allow for real-time data collection and increased automation.
- Enhanced Data Analytics: Advanced analytics tools will enable deeper insights into operational efficiencies and crop production.
- Collaboration Across Sectors: Enhanced collaboration among companies, researchers, and farmers will lead to sharing best practices and data.
- Machine Learning Innovation: Continuous advancements in machine learning algorithms will improve predictive capabilities further.
Conclusion
In summary, the emergence of agriculture datasets for machine learning is revolutionizing how agricultural businesses operate. As data becomes more integral to decision-making processes, understanding and utilizing this data will be crucial in staying competitive. By embracing these technologies, businesses can optimize their practices, enhance productivity, and contribute to sustainable agricultural practices that not only benefit their operations but also the environment.
Investing in effective data management strategies and robust machine learning applications can help agricultural businesses thrive in a rapidly changing marketplace. At Keymakr, we specialize in software development that leverages the power of data, ensuring that businesses in the agriculture sector can achieve their full potential.
agriculture dataset for machine learning